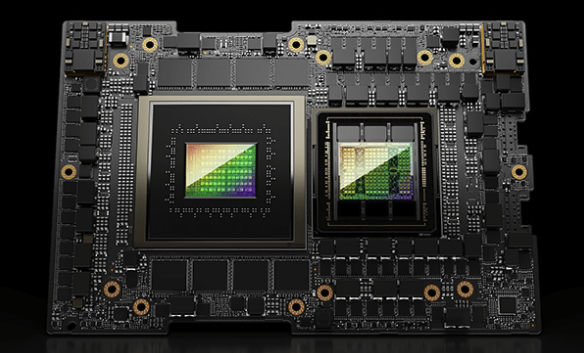
Summary
Recent FY25 capex projections for leading tech giants reveal substantial investments aimed at reinforcing AI data centers:
Amazon: ~$105 billion
Meta: ~$63 billion
Alphabet: ~$75 billion
Microsoft: ~$85 billionThese investments emphasize the dominance of U.S.-based compute clusters as the cornerstone of AI innovation.
While algorithmic advances, such as DeepSeek-R1’s efficient training model (costing approximately $5.58 million for its V3 iteration), highlight emerging trends in AI, U.S. compute clusters maintain an overwhelming advantage. Data from Top500 indicates that the U.S. holds a 55.3% global performance share in supercomputing compared to China's 2.7%.
Despite concerns about future GPU demand, Nvidia remains well-positioned for near-term growth with a projected ~50% one-year return target.
Capex Analysis
Investment Breakdown
Amazon (AMZN): Estimated capex of ~$105 billion for FY25, up from $78 billion in FY24.
Meta (META): Projected to spend ~$63 billion in FY25, compared to $37 billion in FY24.
Alphabet (GOOGL): Expected capex of ~$75 billion, up from $53 billion in FY24.
Microsoft (MSFT): Estimated at ~$85 billion for FY25, up from $44 billion the previous year.
The majority of these expenditures are allocated to AI infrastructure, particularly the development of advanced data centers.
Data Centers vs. Algorithmic Efficiency
Despite the innovation demonstrated by DeepSeek’s algorithmic efficiency, compute clusters remain the central element of AI infrastructure. DeepSeek-R1’s training cost of ~$5.58 million underscores impressive algorithmic gains but does not diminish the foundational importance of data centers. Without access to massive computational resources, even advanced training techniques face limitations.
The reliance on U.S.-based data centers as the dominant AI engine becomes evident when analyzing supercomputer performance data:
System Share: U.S. at 34.6%, China at 12.6%
Performance Share: U.S. leads with 55.3%, while China lags at 2.7%
China’s inability to close this gap reflects its ongoing challenges in semiconductor technology, which remains about a decade behind the U.S.
Implications of DeepSeek-R1
DeepSeek’s ability to achieve low-cost training through efficient algorithms presents a potential shift in the AI landscape. Open-source initiatives like Hugging Face are already attempting to replicate DeepSeek-R1’s model. Big Tech companies, including Meta, have established dedicated teams (such as four "DeepSeek war rooms") to address the emerging competitive threat.
However, lower GPU usage is not an immediate concern:
Short-Term Implications: AI infrastructure expansion continues unabated as Big Tech strives to maintain competitive advantages.
Medium to Long-Term Risks: Efficient training architectures could eventually reduce the demand for GPUs, presenting a challenge for Nvidia.
Despite this risk, Nvidia’s forward-thinking product development—including the Grace Hopper Superchip—positions it to adapt to evolving market demands.
Capex Counter-Analysis
The bearish argument suggests that GPUs will rapidly lose relevance due to algorithmic efficiency. However, FY25 capex estimates from major players (~$300 billion combined) demonstrate sustained investments in compute infrastructure.
Key considerations include:
Diminishing Returns: Deploying excessive GPUs for models trained using efficient algorithms may not yield proportional performance gains.
Specialized Hardware Accelerators: Nvidia’s agile product development strategy suggests it will likely capture new markets as AI training paradigms evolve.
Valuation
Using a conservative terminal P/E multiple of 45 and a discount rate of 18.655% (based on a 4.495% 10-Year Treasury Rate and Nvidia’s beta of 2.36), Nvidia’s one-year return potential remains highly appealing. A base-case outcome suggests a ~50% return over the next year.
Conclusion
Nvidia remains a strong buy despite the potential long-term implications of efficient training architectures.
Key takeaways:
The AI moat remains firmly rooted in compute clusters, where the U.S. holds a dominant position.
DeepSeek-R1’s efficient training innovation is notable but not a threat to Nvidia’s near-term prospects.
Sustained investments in AI infrastructure by Big Tech underscore continued demand for GPUs.
Nvidia’s proactive development of products like the Grace Hopper Superchip positions it to adapt to future market demands.
While a long-term moderation in GPU demand is possible, Nvidia’s innovative capacity and current market positioning suggest that its share price remains secure and poised for growth in the short to medium term.
コメント